Update June, 6, 2022: Diio Signals is no longer being offered by Cirium. Please see Diio Traffic and Fares or DemandView traveler search data for forecasting solutions.
As the travel industry begins to recover in the wake of the COVID-19 pandemic, traditional schedule models need to be supplemented with trusted forward-looking data. Though schedules traditionally have been built by looking backwards and making adjustments, today, accurate scheduling requires airlines to also look forward and make projections without relying on historical reference. The only way to do this Is to incorporate previously hard to digest information (sparse or large data sets), which can be made accessible through applying machine learning techniques.
Cirium has launched its Diio Signals module, which works in conjunction with Diio Mi and SRS Analyzer to provide demand indicators that will help airlines and airports with more effective planning. Diio Signals uses machine learning (ML) to analyze external factors – in this case, corporate events – to determine what type of impact those events will have on a route or destination. It also serves as a proxy to understand corporate travel behavior.
In Cirium’s recent webinar Next Level Airline Decision Systems, Abheer Kolhatkar, product director at Cirium and lead on Cirium’s machine learning products, joined by Matteo Pallini, lead machine learning engineer, and Stephen Quirke, lead product manager, to discuss the intersection of technology and airline decision systems.
What is machine learning and why is it important to the airline industry?
Diio Signals is built by processing un-structured data to identify patterns and generate value for Cirium customers, primarily through the use of machine learning.
Fundamentally, machine learning is focused on identifying patterns in data, being able to learn those patterns and infer what results should be expected, given a specific context.
Matteo Pallini, lead machine learning engineer
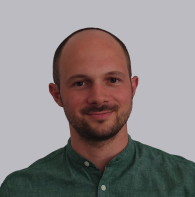
Machine learning has seen massive hype over the last five years as data becomes more and more valuable to global businesses. However, despite a few large tech companies with huge amounts of data making waves in this space, there hasn’t been an incredible amount of meaningful industry change.
Kolhatkar believes the first step towards making machine learning useful for airlines’ daily decision setups in operations, revenue management, marketing sales and so on, is for companies to spend time making this data available.
We’re starting to see a lot of middleware companies who take mundane and human-heavy tasks airlines rely on and simplifying them, making them quicker with better accuracy. Hopefully airlines will be one of the next players to win from this approach.
Abheer Kolhatkar, product director
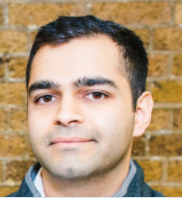
Some might perceive machine learning as a threat, but Pallini explains why the airline industry should focus on the positives that ML can offer. Where operational learnings within an organization are held by humans, if those humans leave, you would have had to start again to rebuild the knowledge base. If it’s embedded in the system, it can’t be lost. ‘The way I see it, any automation is more likely to automate the things that are more mundane to do, leaving more time to focus on added-value tasks.’ In short, machine learning should lift all ships.
Working with data in a volatile world
At present, the aviation industry hasn’t fully embraced machine learning because it hasn’t needed to. ‘Rule-based systems, user influence and user input were always king,’ Kolhatkar continues. ‘Things were fairly static, so you could use last year’s data and increment it in some meaningful way and be accurate enough.’ Yet, in a post-COVID world, a reduction in airline staffing has led to decision bottlenecks of too much information and too little time. This volatility has led to an increase in the amount of decisions which airlines need to make under tight time pressures with fewer data points.
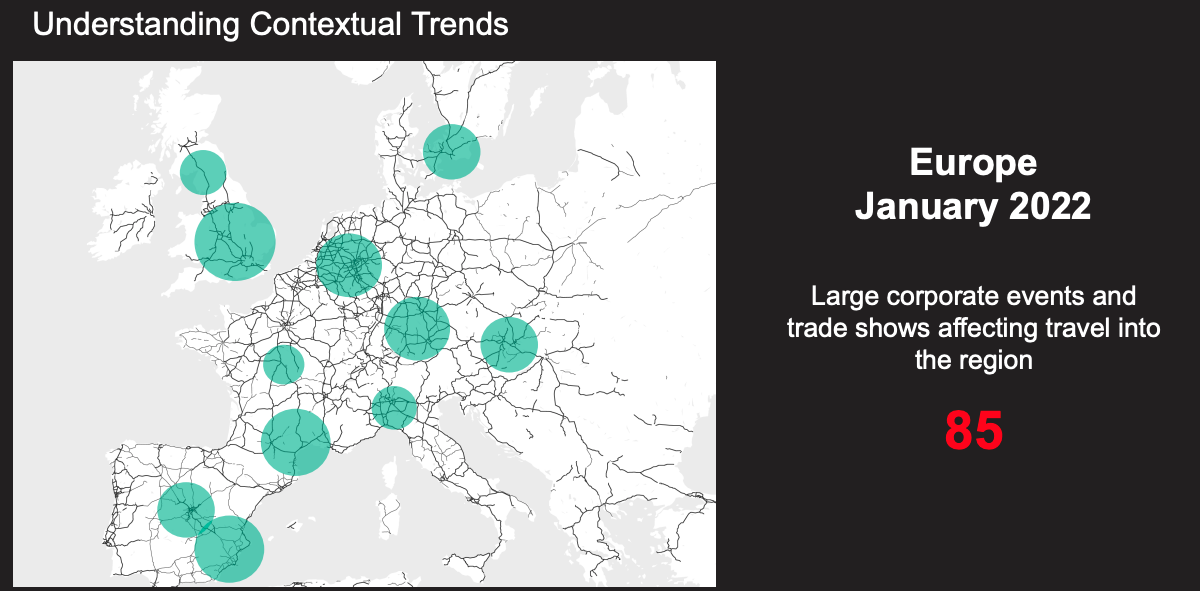
Pallini believes that this volatility has also been influenced by generational behavioral changes. ‘Travelers have become more fluid in the last 10-15 years. My parents used to go to the same places every year, whereas my generation is more interested in trying something new almost every year, COVID-allowing. This is why it’s important to consider contextual data and non-traditional data sources, which is much easier to do if you use machine learning.’
Where passenger demand modelling has been a prime opportunity for the travel sector and the airline sector, in a post-COVID world, predicting passenger demand has become a huge challenge. ‘Volatility has changed a lot,’ admits Kolhatkar. ‘People are choosing to book last minute and being more erratic about where they are choosing to go. This is partially influenced by the environment – Where can they go? Where is it safe to go? – but also they’re making decisions around pricing availability, convenience, etc.
If the world is driven much more by the environment, then understanding the environment becomes the core task. But it’s hard to do that, hence the need for ML.
Abheer Kolhatkar, product director
Using UK-based traveler sentiment towards Spain as an example, Kolhatkar explains that using last year’s data doesn’t really help answer the question. ‘You can look at restrictions, you can look at consumer sentiment… and once you understand these biases that people build up, it becomes a very interesting machine learning problem.’
Taking all these data requirements into consideration, Pallini asserts that a paradigm shift needs to take place in the industry, where value is placed on the quality of data, rather than new technology or tools.
Data should be treated more as an asset than as a byproduct of business as usual. The more we shift towards that kind of culture and the easier we make it for analysts, data scientists, anyone within the company to access the data, the more we will be able to open ourselves up to opportunities.
Matteo Pallini, lead machine learning engineer
Many have questioned whether using current or pre-COVID data is useful in terms of plotting recovery and forecasting for the future, but Pallini believes that pre-COVID data is still useful in terms of relative magnitude. Events in major financial hubs such as New York, London, Barcelona and Berlin will still see interest and attract visitors from other major financial hubs. ‘However, restrictions, number of cases and fear have significantly changed how people decide to travel, so the most recent data is the only good source we have to get an understanding of how these factors play a role. We can’t call it bad data because as long as COVID-19 is a problem and restrictions are in place, current data is giving us more signals about how people decide to travel and what is going to happen in the next six months, than the data we had before COVID-19. I would discard data from the first three or four months of the pandemic in 2020, but everything after that is useful.’
Using Diio Signals to analyze corporate event travel
Given that the aim of Diio Signals is to use data to answer evergreen questions, for this iteration the team started with the following principles:
- Is there a way to meaningfully estimate how people are going to travel for events?
- Can we say exactly on which route people are going to travel for an event and give an estimate of how many passengers are going from A to B, and the distribution?
- How does it change over time?
If the fundamental question is ‘will people travel for events?’ The answer is mostly yes. Even if it’s somewhat scaling up and down as restrictions lift. From there, the next question is: if we incorporate restrictions, how are people going to travel for events? That is what Diio Signals aims to answer.
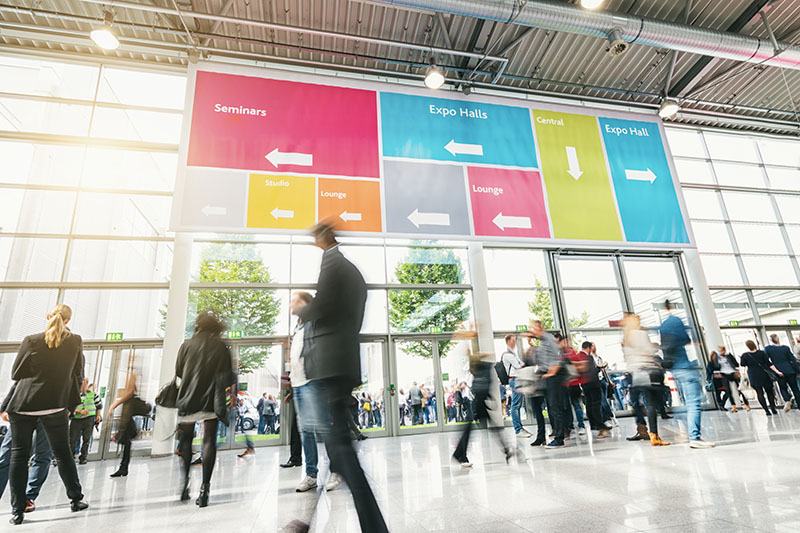
‘As people start flying again, the signal will still be useful post-COVID. If you had 10 or 20 events in 2019 that attracted a lot of people, those events are going to come back and they’re still going to be popular,’ Kolhatkar explains. ‘It makes sense to track these events because they come at the start of the sales funnel. By looking at the signal, we can start understanding where corporate travelers are going to meet their customers and can estimate and forecast where they are going to come from to do so. If you know where people are travelling from, you have more information around how you build your networks, how you plan for people coming in and how you want to serve them. That’s valuable to have.’
The team is utilizing contextual demand insights, including search and booking data, events data and news data to find out which events are popular, which events people are going to travel for and how many people will travel to that event from a variety of single origins. Which will in turn affect network planning and revenue management, rebuilding confidence back into these systems.
The importance of contextual insights
As discussed earlier in this article, environmental factors are crucial to consider in an increasingly changing world. As Kolhatkar explains, ‘These factors matter – and they’re going to matter more, especially when someone is working to attract customers and you’re not meeting that demand. To me, context is environmental insights. It’s what you don’t answer with current industry data and what can we bring in to answer those questions. It’s never going to be 100% accurate, but it’s going to maybe answer the last 20% of uncertainty around certain questions we have, and that’s pretty good.’
We’re hoping to bridge the gap between data that airlines currently have and the questions which are left unanswered. Understanding corporate travel behavior is an important question for airlines to answer. It’s arguably an important question for almost every single person in the travel sector to answer, because it drives a lot of revenue for the sector.
Abheer Kolhatkar, product director
‘Even if Diio Signals makes a 5% improvement in decision-making around how to price inventory, how to allocate aircraft, how many business-class seats to put on a route, we will be very happy.’
What data sources does the first iteration use?
What data should make up part of the first data set? News data offers certain insights into customer sentiment, highlighting how often a London news outlet talks about Indonesia, for example, or how many bloggers mention Bali. But this is just the tip of the iceberg. Kolhatkar continues, ‘We use as much public, non-private information as possible, including news and completely PII-removed data sets because we don’t like to share personal information. From a pipeline perspective, we also have the benefit of companies under the RELX brand umbrella. We have the best news aggregator in the world, a sister company of ours, as well as the biggest business event organizer.
‘If you start connecting these dots with enough places you can start building a travel trend index that says what’s popular. Where are people really interested in going? What are they talking about right now? And how does this correlate with what you’ve observed in the past?’ Another approach is to look at search and book data which Kolhatkar states ‘is a great place to get early intuitions about what people actually care about today. Broadly, we’re trying to answer, on a slice-by-slice basis, how consumers are behaving through what we’re observing online.’
What’s next for Diio Signals?
With the first dataset generating industry buzz as we speak, the next iteration, which is already in the works, will focus on leisure travel trends. Look out for its launch during Q2 of 2022.
To watch the Next Level Airline Decision Systems webinar click here.
Or learn more about Diio Signals and schedule a demo.